Lost in the details? 4 simple steps to help students better understand study results.
Posted January 12, 2016
By David Disabato
You know how it is: your instructor assigns you to read a study and things go along well until you hit that dreaded results section. Suddenly, the English language is replaced with numbers, Greek symbols, and asterisks. They mean something; but unless you have a background in statistics you might not always know exactly what. Let’s be honest; confusion over results of empirical articles motivates many students to skip over these sections entirely. My goal with this post is to help you engage with these tough articles by explaining what statistics in psychology can and cannot do.
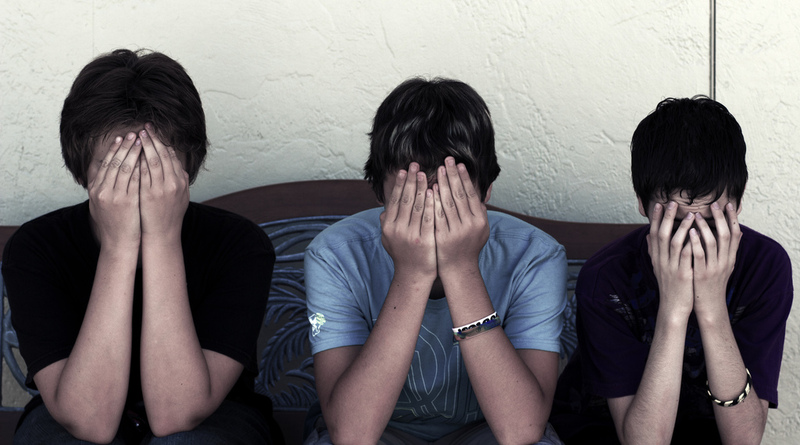
What statistics CAN do:
Statistics can determine whether variables are correlated. Correlation is a measure of the relationship between two variables. You are probably most familiar with the term “correlation” from your statistics class when you calculated “r” ranging from -1 to +1. Although other statistics have different symbols (e.g., t, F, d, which you may or may not remember from statistics class), they are actually all different types of correlations. Now most of you are probably comfortable reading results sections with the “r” correlation – perhaps that is the only statistic you understand! The key is realizing more advanced statistics are just fancy correlations. When you see big tables, with many columns, numbers, and asterisks, or figures with circles, boxes and arrows, they are almost always representing correlations of some sort.
What stats CANNOT do:
Statistics can never determine causation. Remember the old saying: “Correlation does not equal causation?” Maybe you learned that the amount of ice cream sales at a beach is correlated with the number of shark attacks in the water. But it would be unlikely that ice cream sales cause shark attacks. Variables can be correlated for many reasons other than one variable causing the other. This logic applies to not only the “r” correlation statistic, but all other advanced statistics as well. No matter how complex the statistics, they only determine correlation and not causation.
What DOES determine causation?
Causation is determined by the research design reported in the methods section, not the statistics found in the results section. Remember learning about all the different types of research designs: true experiments, quasi-experiments, observational studies, survey studies, case studies, etc. Well only true experiments are able to determine causation and all other study designs are not able to. That is why your professors may have emphasized the scientific power of true experiments (see http://psychology.ucdavis.edu/faculty_sites/sommerb/sommerdemo/experiment/types.htm for a good definition of a true experiment).
HOW to read a results section:
When reading a results section, one of the goals is to decide whether there is enough evidence to support the researcher’s claims in the discussion and abstract sections. Below are four steps I believe are central to reading a results section. To help guide you through the process – let’s use an example: A study wants to see whether a college students’ number of friends is associated with greater alcohol use.
1) Determine the variable thought to be the cause. In our example, the variable is “number of friends”.
2) Determine the variable thought to be the effect. In our example, the variable is “alcohol use”.
3) Determine which statistic represents the “correlation” between the two variables. Remember, almost every statistic represents a correlation, but the symbol may be different than the traditional “r”. In our example, the correlation may come from a regression with a “t” statistic.
4) Determine whether the correlation between the two variables is statistically significant. This is just a fancy way of looking at probability. Basically, researchers are asking, “What is the probability the correlation was due to random chance?”Remember that thing called a “p-value” from statistics class? (P.S., lower is better). If the p-value associated with the statistic is less than .05 (e.g., p < .05), then the correlation is “statistically significant”, implying a correlation exists. If the correlation is not statistically significant, the two variables are said to be uncorrelated. In our example, we will assume statistical significance.
Although our example only has one variable thought to be the cause and one the effect, complex studies often have multiple “causes” and “effects”. No matter how many, we can apply the same four steps to each combination of thought to be cause-effect pairs.
Hopefully we succeed in identifying the key words, numbers, and symbols in the results section to complete steps 1-3. Step 4 will determine whether the researcher’s claim is supported or not. However, be weary of seemingly causal language in the discussion and abstract sections with phrases such as “predicts”, “leads to”, “influences”, “affects”, etc. These are some of the many ways researchers write about their statistical correlations, but the words do not change the fact that “correlation does not equal causation”.
SUMMARY of what we learned:
We have learned what statistics can do: determine whether two variables are correlated. We learned what statistics cannot do: determine whether one variable causes the other. We learned that the type or research design, not the statistic, determines causation. We learned the four steps of evaluating whether the results support the researcher’s claims in the discussion and abstract sections: 1) Identify the variable(s) thought to be the cause, 2) Identify the variable(s) thought to be the effect, 3) Identify the statistic representing their correlation, and 4) determine whether that correlation is statistically significant.
Don’t let results sections intimidate you. All studies are simply researchers asking questions about interesting variables. They usually want to know the relationship between variables and in some cases, the relationship over time. In some cases they can determine if one variable causes another. Parsing apart each variable and understanding whether results are significant is well within your grasp.
Bio
David Disabato is a PhD student of Clinical Psychology at George Mason University outside of Washington DC. He specializes in statistics and data analysis as it applies to non-experimental psychology. He has done statistical consulting for multiple professors across the country using new, up and coming analyses. He has taught statistics at the undergraduate and graduate levels and is interested in discovering new ways to motivate psychologists to use statistical best practice.