Intellectual Abilities, Interests, and Mastery
By David LubinskiVanderbilt University
Psychologists interested in the study of human individuality have found that accomplishments in education, the world of work, and creativity are a joint function of talent, passion, and commitment — or how much effort and time one is willing to invest in personal development when the opportunity is provided. This module reviews models and measures that psychologists have designed to assess intellect, interests, and energy for personal development. The module begins with a model for organizing these three psychological domains, which is useful for understanding talent development. This model is not only helpful for understanding the many different ways that positive development may unfold among people, but it is also useful for conceptualizing personal development and ways of selecting opportunities in learning and work settings that are more personally meaningful. Data supporting this model are reviewed.
Learning Objectives
- Compare and contrast satisfaction and satisfactoriness.
- Discuss why the model of talent development offered in this module places equal emphasis on assessing the person and assessing the environment.
- Articulate the relationship between ability and learning and performance.
- Understand the issue of an "ability threshold" beyond which more ability may or may not matter.
- List personal attributes other than interests and abilities that are important to individual accomplishment.
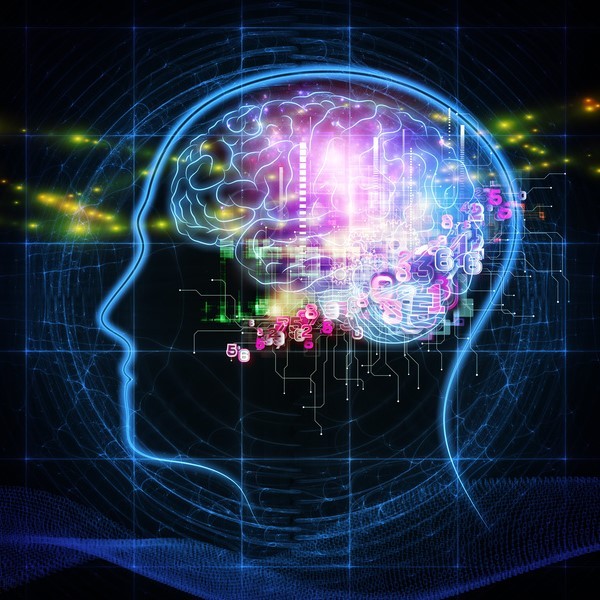
An amalgam of intelligence, interests, and mastery are appropriate topics for an essay on the cross-cutting themes running through these vast domains of psychological diversity. For effective performance and valued accomplishments, these three classes of determinants are needed for comprehensive treatments of psychological phenomena supporting learning, occupational performance, and for advancing knowledge through innovative solutions. Historically, these personal attributes go back to at least Plato’s triarchic view of the human psyche, described in Phaedra, wherein he depicts the intellect as a charioteer, and affect(interests) and will(to master) as horses that draw the chariot. Ever since that time, cognitive, affective, and conative factors have all been found in comprehensive models of human development, or “The Trilogy of Mind” (Hilgard, 1980). To predict the magnitude, nature, and sophistication of intellectual development toward learning, working, and creating, all three classes are indispensable and deficits on any one can markedly hobble the effectiveness of the others in meeting standards for typical as well as extraordinary performance. These three aspects of human individuality all operate in parallel confluences of behaviors, perceptions, and stimuli to engender stream of consciousness experiences as well as effective functioning. Hilgard (1980) was indeed justified to criticize formulations in cognitive psychology, which neglect affection and conation; technically, such truncated frameworks of human psychological phenomena are known as under-determined or misspecified causal models (Lubinski, 2000;Lubinski & Humphreys, 1997).
A Framework for Understanding Talent Development
Figure 1 is an adaptation of the Theory of Work Adjustment (TWA; Dawis & Lofquist, 1984; Lubinski & Benbow, 2000). It provides a useful organizational scheme for this treatment by outlining critical dimensions of human individuality for performance in learning and work settings (and in transitioning between such settings). Here, the dominant models of intellectual abilities and educational–occupational interests are assembled. Because this review will be restricted to measures of individual differences that harbor real-world significance, these two models are linked to corresponding features of learning and work environments, ability requirements and incentive or reward structures, which set standards for meeting expectations (performance) and rewarding valued performance (compensation). Correspondence between abilities and ability requirements constitutes satisfactoriness (“competence”), whereas correspondence between an interests and reward structures constitutes satisfaction (“fulfillment”). To the extent that satisfactoriness and satisfaction co-occur, the individual is motivated to maintain contact with the environment and the environment is motivated to retain the individual; if one of these dimensions is dis-correspondent, the individual is motivated to leave the environment or the environment is motivated to dismiss.
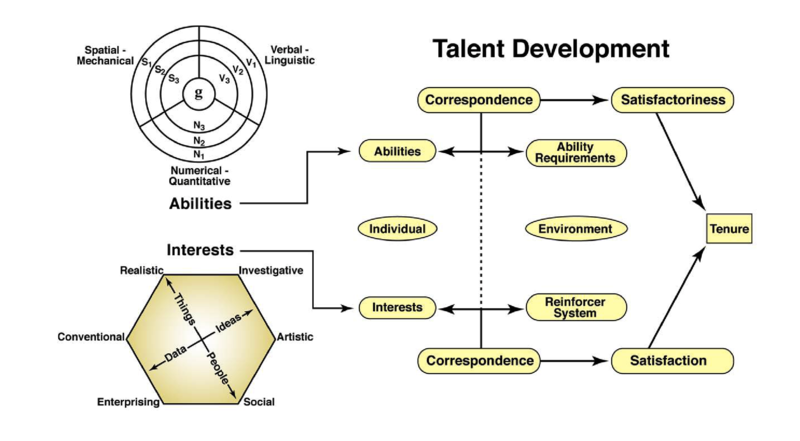
This model of talent development places equal emphasis on assessing the individual (abilities and interests) and the environment (response requirements and reward structures). Comprehensive reviews of outcomes within education (Lubinski, 1996; Lubinski & Benbow, 2000), counseling (Dawis, 1992; Gottfredson, 2003; Rounds & Tracey, 1990), and industrial/organizational psychology all emphasize this person/environment tandem (Dawis, 1991; Katzell, 1994; Lubinski & Dawis, 1992; Strong, 1943): aligning competency/motivational proclivities to performance standards and reward structures for learning and work (Bouchard, 1997; Scarr, 1996; Scarr & McCartney, 1983). And indeed, educational, counseling, and industrial psychology can be contiguously sequenced by this framework. They all share a common feature: the scientific study of implementing interventions or opportunities, based on individual differences, for maximizing positive psychological growth across different stages of life span development (Lubinski, 1996). For making individual decisions about personal development, or institutional decisions about organizational development, it is frequently useful to go beyond a minimum requisite approach of “do you like it” (satisfaction) and “can you do it” (satisfactoriness), and instead consider what individuals like the most and can do the best (Lubinski & Benbow, 2000, 2001). This framework is useful for identifying “optimal promise” for personal as well as organizational development. For now, however, cognitive abilities and interests will be reviewed and, ultimately, linked to conative determinants that mobilize, and in part account for, individual differences in how capabilities and motives are expressed.
Cognitive Abilities
Over the past several decades—the past 20 years in particular—a remarkable consensus has emerged that cognitive abilities are organized hierarchically (Carroll, 1993). A general outline of this hierarchy is represented graphically by a radex (Guttman, 1954), depicted in the upper left region of Figure 1. This illustrates the reliable finding that cognitive ability assessments covary as a function of their content or complexity (Corno, Cronbach et al., 2002; Lubinski & Dawis, 1992; Snow & Lohman, 1989). Cognitive ability tests can be scaled in this space based on how highly they covary with one another. The more that two tests share complexity and content, the more they covary and the closer they are to one another as points within the radex. Test complexity is scaled from the center of the radex (“g”) out, and, along lines emanating from the origin, complexity decreases but test content remains the same. Test content is scaled around the circular bands with equal distance from the center of the radex and, progressing around these bands, the relative density of test content changes from spatial/mechanical to verbal/linguistic to quantitative/numerical, but test complexity remains constant. Therefore, test content varies within each band (but complexity remains constant), whereas test complexity varies between bands (but on lines from the origin to the periphery, content remains constant). Because the extent to which tests covary is represented by how close together they are within this space (Lubinski & Dawis, 1992; Snow & Lohman, 1989; Wai, Lubinski, & Benbow, 2009), this model is helpful in organizing the many different kinds of specific ability tests.
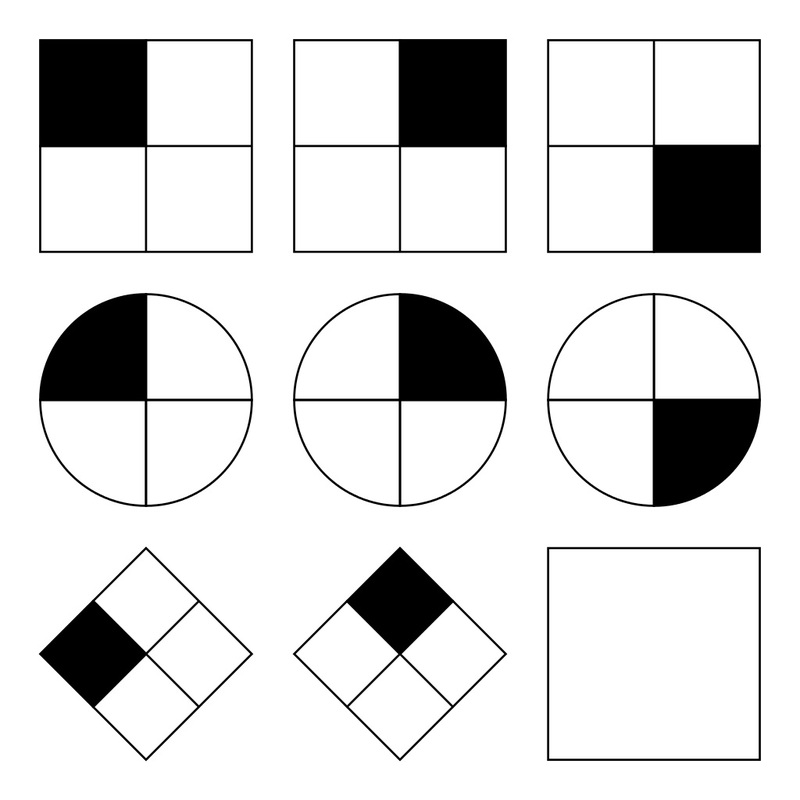
As Piaget astutely pointed out, “Intelligence is what you use when you don’t know what to do,” and this model affords an excellent overview of the content and sophistication of thought applied to familiar and novel problem-solving tasks. Mathematical, spatial, and verbal reasoning constitute the chief specific abilities with implications for different choices and performance after those choices in learning and work settings (Corno et al., 2002; Dawis, 1992; Gottfredson, 2003; Lubinski, 2004; Wai et al., 2009). The content of measures or tests of these specific abilities index individual differences in different modalities of thought: reasoning with numbers, words, and figures or shapes. Yet, despite this disparate content and focus, contrasting specific ability tests are all positively correlated, because they all index an underlying general property of intellectual thought.
This general (common) dimension, identified over 100 years ago (Spearman, 1904) and corroborated by a massive quantity of subsequent research (Carroll, 1993; Jensen, 1998), is general mental ability, the general factor, or simply g (Gottfredson, 1997). General mental ability represents the complexity/sophistication of a person’s intellectual repertoire (Jensen, 1998; Lubinski & Dawis, 1992). The more complex a test is, regardless of its content, the better a measure of g it is. Further, because g underlies all cognitive reasoning processes, any test that assesses a specific ability is also, to some extent, a measure of g (Lubinski, 2004). In school, work, and a variety of everyday life circumstances, assessments of this general dimension covary more broadly and deeper than any other measure of human individuality (Hunt, 2011; Jensen, 1998; Lubinski, 2000, 2004).
Measures of g manifest their life importance by going beyond educational settings (where they covary with educational achievement assessments in the .70–.80 range), by playing a role in shaping phenomena within Freud’s two important life domains, arbeiten and lieben, working and loving (or, resource acquisition and mating). Measures of g covary .20–.60 with work performance as a function of job complexity, .30–.40 with income, and –.20 with criminal behavior, .40 with SES of origin, and .50–.70 with achieved SES; assortative mating correlations on g are around .50 (Jensen, 1998; Lubinski, 2004; Schmidt & Hunter, 1998). Furthermore, Malcolm Gladwell (2008) notwithstanding, there does not appear to be an ability threshold; that is, the idea that after a certain point more ability does not matter. More ability does matter.
Although other determinants are certainly needed (interests, persistence, opportunity), more ability does make a difference in learning, working, and creating, even among the top 1% of ability, or IQ equivalents ranging from approximately 137 to over 200 (see Figure 2). When appropriate assessment and criterion measures are utilized to capture the breadth of ability and accomplishment differences among the profoundly talented, individual differences within the top 1% of ability are shown to matter a great deal. In the past this has been difficult to demonstrate, because intellectual assessments and criterion measures lacked sufficient scope in gifted or intellectually talented populations, which resulted in no variation in assessments among the able and exceptionally able (ceiling effects). Without variation there cannot be co-variation, but modern methods have now corrected for this (Kell, Lubinski, & Benbow, 2013a; Lubinski, 2009; Park, Lubinski, & Benbow, 2007, 2008). Yet, even when g is measured in its full scope, and validated with large samples and appropriate low-base-rate-criteria over protracted longitudinal intervals, there is much more to intellectual functioning than measures of g or general ability.
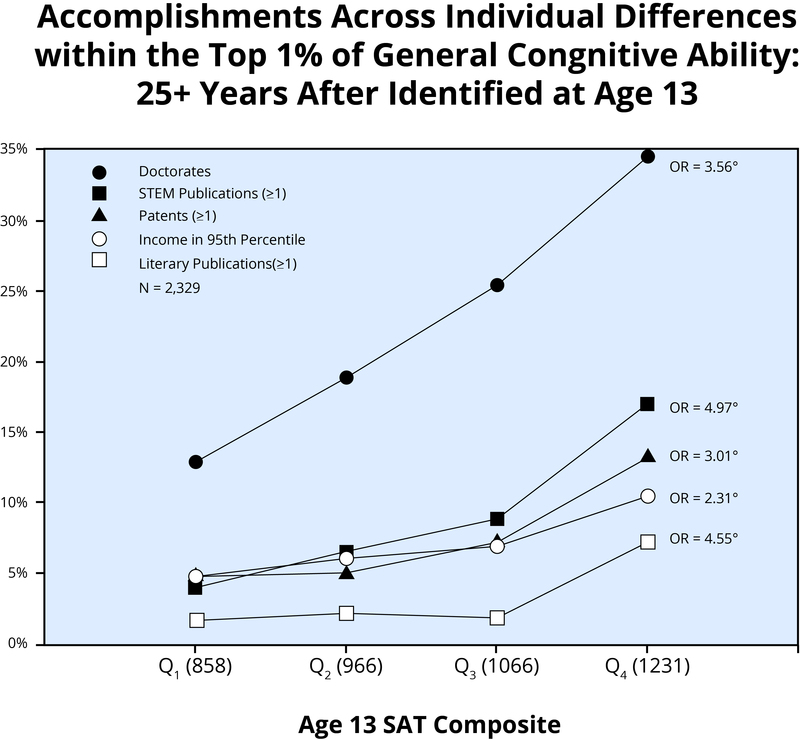
To reveal how general and specific abilities operate over the course of development, Figure 3 contains data from over 400,000 high schools students assessed between grades 9 through 12, and tracked for 11 years. Specifically, Figure 3 graphs the general and specific ability profiles of students earning terminal degrees in nine disciplines (Wai et al., 2009). Given that highly congruent findings were observed for all four cohorts (grades 9 through 12), the cohorts were combined. High general intelligence and an intellectual orientation dominated by high mathematical and spatial abilities, relative to verbal ability, were salient characteristics of individuals who pursued advanced education credentials in science, technology, engineering, and mathematics (STEM). These participants occupy a region in the intellectual space defined by the dimensions of ability level and ability pattern different from participants who earn undergraduate and graduate degrees in other domains.
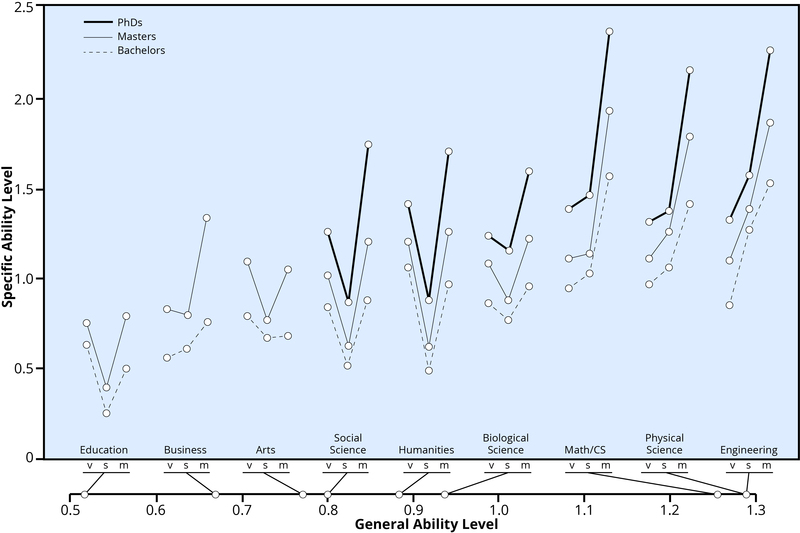
Two major differences distinguish the STEM from the non-STEM educational groups. First, students who ultimately secure educational credentials in STEM domains are more capable than those earning degrees in other areas, especially in nonverbal intellectual abilities. Within all educational domains, more advanced degrees are associated with more general and specific abilities. Second, for all three STEM educational groupings (and the advanced degrees within these groupings), spatial ability > verbal ability—whereas for all others, ranging from education to biology, spatial ability < verbal ability (with business being an exception). Young adolescents who subsequently secured advanced educational credentials in STEM manifested a spatial–verbal ability pattern opposite that of those who ultimately earned educational credentials in other areas. These same patterns play out in occupational arenas in predictable ways (Kell, Lubinski, Benbow, & Steiger, 2013b). In the past decade, individual differences within the top 1% of ability have revealed that these patterns portend important outcomes for technical innovation and creativity, with respect to both ability level (Lubinski, 2009; Park et al., 2008) and pattern (Kell et al. 2013a, Kell et al., 2013b; Park et al., 2007). Level of general ability has predictive validity for the magnitude of accomplishment (how extraordinary they are), whereas ability pattern has predictive validity for the nature of accomplishments (the domains they occur in).
Interests
Just because people can do something well doesn’t mean they like doing it. Psychological information on motivational differences (personal passions) is needed to understand attractions and aversions, different ways to create a meaningful life, and how differential development unfolds. Even people with the same intellectual equipment vary widely in their motivational proclivities. Paraphrasing Plato, different horses drive intellectual development down different life paths. The lower left region of Figure 1 provides the dominant model of vocational interests, one developed from decades of large-scale longitudinal and cross-cultural research. It shows a hexagonal structure consisting of six general themes: Realistic (R) = working with gadgets and things, the outdoors, need for structure; Investigative (I) = scientific pursuits, especially mathematics and the physical science, an interest in theory; Artistic (A) = creative expression in art and writing, little need for structure; Social (S) = people interests, the helping professions, teaching, nursing, counseling; Enterprising (E) = likes leadership roles directed toward economic objectives; and Conventional (C) = liking of well-structured environments and clear chains of command, such as office practices.
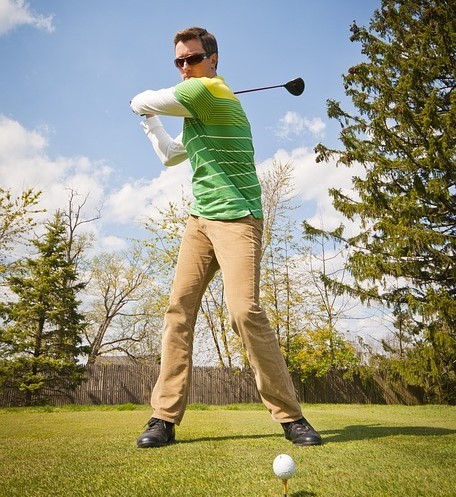
These six themes covary inversely with the distance between them, hence, the hexagonal structure circling around R-I-A-S-E-C. John Holland (1959, 1996) justifiably receives most of the credit for this model (Day & Rounds, 1998), although Guilford et al. (1954) uncovered a similar framework based on military data and labeled them Mechanical, Scientific, Aesthetic Expression, Social Welfare, Business, and Clerical. Although each theme contains multiple subcomponents, Holland’s hexagon, like the radex of cognitive abilities, captures the general outlines of the educational/occupational interest domain, but there are molecular strands of intellective and interest dimensions that add nuance to these general outlines (for abilities, see Carroll, 1993; for interests, see Dawis, 1991; Savickas & Spokane, 1999). There are also super-ordinal themes such as people versus things (Su, Rounds, & Armstrong, 2009), which manifest arguably the largest sex-difference on a psychological dimension of human individuality.
At superordinate levels of people versus things or data versus ideas (Prediger, 1982), or at the RIASEC level of analysis, interest dimensions covary in different ways with mathematical, spatial, and verbal abilities (Ackerman, 1996; Ackerman & Heggestad, 1997; Schmidt, Lubinski, & Benbow, 1998); and intense selection, when exclusively restricted to a specific ability, will eventuate in distinctive interest profiles across the three abilities with implications for differential development (Humphreys, Lubinski, & Yao, 1993; Webb, Lubinski, & Benbow, 2007). Although correlations between abilities and interests are “only” in the .20–.30 range, when selection is extreme, distinct profiles emerge and reflect different “types” (Lubinski & Benbow, 2000, 2006). For basic science, this shows how ostensibly different kinds of intelligence at the extreme do not stem from different qualities, but rather from endpoint extremes within a multivariate space of systematic sources of individual differences, which “pull” with them constellations of nonintellectual personal attributes. For applied practice, skilled educational–vocational counselors routinely combine information on abilities and interests to distill learning and work environments that individuals are likely to thrive in competence and experience fulfillment (Dawis, 1992; Rounds & Tracy, 1990). For further insights, a final class of important psychological determinants is needed, however.
Mastery
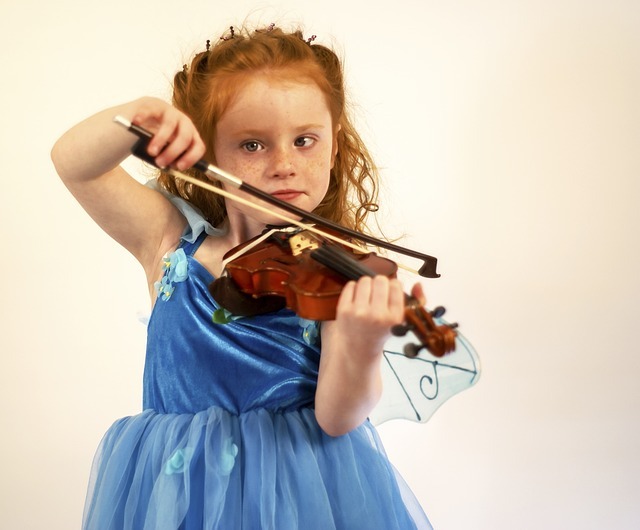
As all parents of more than one child know, there are huge individual differences in the extent to which individuals embrace opportunities for positive development. Seasoned faculty at top institutions for graduate training have observed the same phenonemon—among highly select graduate students, task commitment varies tremendously. Even among the intellectual elite, individual differences in accomplishments stem from more than abilities, interests, and opportunity; conative determinants are critical catalysts. Galton (1869) called it “zeal,” Hull (1928) called it “industriousness,” and Webb (1915) called it “will.” Such labels as “grit” or “strivers” are sometimes used to define resources that people call upon to mobilize their abilities and interests over protracted intervals. Conative factors are distinct from abilities and preferences, having more to do with individual differences in energy or psychological tempo rather than the content of what people can do or how rapidly they learn. Indeed, characteristic across scientific studies of expertise and world-class accomplishment are attributes specifically indicative of indefatigable capacities for study and work. This is an underappreciated class of individual differences, although Ackerman (1996) has discussed typical intellectual engagement (TIE) and Dawis and Lofquist (1984) have discussed pace and endurance. This class of attributes simply has not received the attention it deserves.
Nevertheless, in the field of talent development and identification, the greatest consensus appears to be found on the topic of conation, rather than cognition or affect. Exceptional performers are deeply committed to what they do, and they devote a great deal of time to doing it. Regardless of the theorist, Howard Gardner, Dean Simonton, Arthur Jensen, Anders Erikson, and Harriet Zuckerman all agree that this is a uniform characteristic of world class performers at the top of their game. In the words of Dean Simonton and E. O. Wilson, respectively:
[M]aking it big [becoming a star] is a career. People who wish to do so must organize their whole lives around a single enterprise. They must be monomaniacs, even megalomaniacs, about their pursuits. They must start early, labor continuously, and never give up the cause. Success is not for the lazy, procrastinating, or mercurial. (Simonton, 1994, p. 181)
I have been presumptuous enough to counsel new Ph.D.’s in biology as follows: If you choose an academic career you will need forty hours a week to perform teaching and administrative duties, another twenty hours on top of that to conduct respectable research, and still another twenty hours to accomplish really important research. This formula is not boot-camp rhetoric. (Wilson, 1998, pp. 55–56)
Figure 4 contains data from two extraordinary populations of individuals (Lubinski, Benbow, Webb, Bleske-Rechek, 2006). One group consists of a sample of profoundly gifted adolescents identified at age 12 as in the top 1 in 10,000 in mathematical or verbal reasoning ability; they were subsequently tracked for 20 years. Members of the second group were identified in their early twenties, as first- or second-year STEM graduate students enrolled in a top-15 U.S. university; they were subsequently tracked for 10 years. Now in their mid-thirties, subjects were asked how much they would be willing to work in their “ideal job” and, second, how much they actually do work. The data are clear. There are huge individual differences associated with how much time people are willing to invest in their career development and work. The STEM graduate students are particularly interesting inasmuch as in their mid-twenties they were assessed on abilities, interests, and personality, and both sexes were found to be highly similar on these psychological dimensions (Lubinski, Benbow, Shea, Eftekhari-Sanjani, & Halvorson, 2001). But subsequently, over the life span, they markedly diverged in time allocation and life priorities (Ceci & Williams, 2011; Ferriman, Lubinski, & Benbow, 2009).
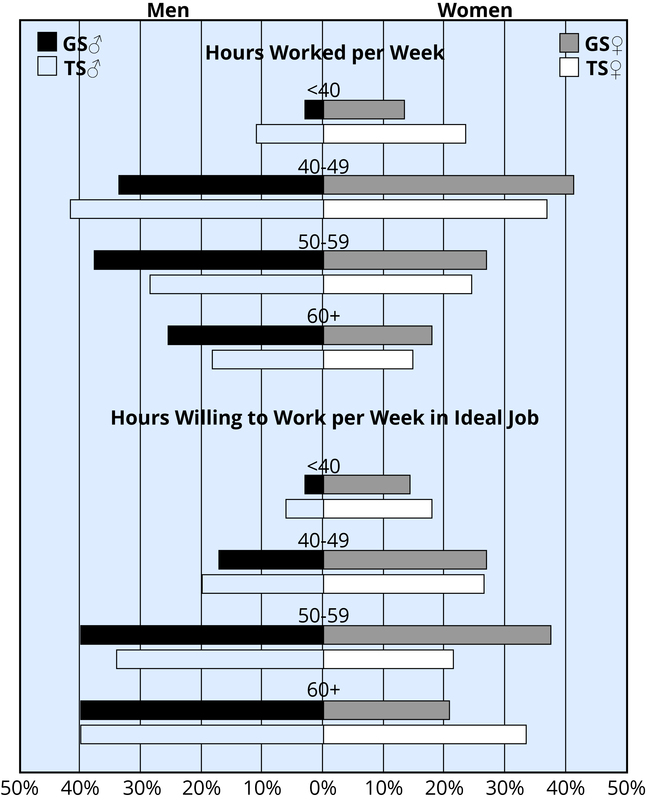
These figures reveal huge noncognitive individual differences among individuals with exceptional intellectual talent. One only needs to imagine the ticking of a tenure clock and the differences likely to accrue over a 5-year interval between two faculty working 45- versus 65-hour weeks (other things being equal). Making partner in a prestigious law firm is no different, nor is achieving genuine excellence in most intellectually demanding areas.
Conclusion
Since Spearman (1904) advanced the idea of general intelligence, a steady stream of systematic scientific knowledge has accrued in the psychological study of human individuality. We have learned that the intellect is organized hierarchically, that interests are multidimensional and only covary slightly with abilities, and that individual differences are huge in terms of investing in personal development. When these aspects of human psychological diversity are combined with commensurate attention devoted to opportunities for learning, work, and personal growth, a framework for understanding human development begins to take shape. Because frameworks may be found that emphasize only one set of these determinants, this essay closes with the recommendation—based on the empirical evidence—to stress all three.
Outside Resources
- Book: Human Cognitive Abilities, by John Carroll constitutes a definitive treatment of the nature and hierarchical organization of cognitive abilities, based on a conceptual and empirical analysis of the past century’s factor analytic research.
- http://www.amazon.com/Human-Cognitive-Abilities-Factor-Analytic-Studies/dp/0521382750/ref=sr_1_1?s=books&ie=UTF8&qid=1390944516&sr=1-1&keywords=human+cognitive+abilities
- Book: Human Intelligence, by Earl Hunt, provides a superb overview of empirical research on cognitive abilities. Collectively, these three sources capture the psychological significance of what this important domain of human psychological diversity affords.
- http://www.amazon.com/Human-Intelligence-Earl-Hunt/dp/0521707811
- Book: The g Factor, by Arthur Jensen, explicates the depth and breadth of the central dimension running through all cognitive abilities, the summit of Carroll’s (1993) hierarchical organization: general intelligence (or “g”). Revealed here is the practical and scientific significance for coming to terms with a rich array of critical human outcomes found in schools, work, and everyday life.
- http://www.amazon.com/The-Factor-Evolution-Behavior-Intelligence/dp/0275961036
- Book: For additional reading on the history of intellectual assessment, read Century of Ability Testing, by Robert Thorndike and David F. Lohman
- http://www.amazon.com/Century-Ability-Testing-Robert-Thorndike/dp/0829251561
Discussion Questions
- Why are abilities and interests insufficient for conceptualizing educational and occupational development?
- Why does the model of talent development discussed in this module place equal emphasis on assessing the individual and assessing the environment.
- What is the most widely agreed on empirical finding, among investigators who study the development of truly outstanding careers?
- Besides what you can do and what you like, what other factors are important to consider when making choices about your personal development in learning and work settings?
Vocabulary
- g or general mental ability
- The general factor common to all cognitive ability measures, “a very general mental capacity that, among other things, involves the ability to reason, plan, solve problems, think abstractly, comprehend complex ideas, learn quickly, and learn from experience. It is not merely book learning, a narrow academic skill, or test-taking smarts. Rather, it reflects a broader and deeper capability for comprehending our surroundings—‘catching on,’ ‘making sense of things,’ or ‘figuring out’ what to do” (Gottfredson, 1997, p. 13).
- Satisfaction
- Correspondence between an individual’s needs or preferences and the rewards offered by the environment.
- Satisfactoriness
- Correspondence between an individual’s abilities and the ability requirements of the environment.
- Specific abilities
- Cognitive abilities that contain an appreciable component of g or general ability, but also contain a large component of a more content-focused talent such as mathematical, spatial, or verbal ability; patterns of specific abilities channel development down different paths as a function of an individual’s relative strengths and weaknesses.
- Under-determined or misspecified causal models
- Psychological frameworks that miss or neglect to include one or more of the critical determinants of the phenomenon under analysis.
References
- Ackerman, P. L. (1996). A theory of adult intellectual development: Process, personality, interests, and knowledge. Intelligence, 22, 227–257.
- Ackerman, P. L., & Heggestad, E. D. (1997). Intelligence, personality, and interests: Evidence for overlapping traits. Psychological Bulletin, 121, 218–245.
- Bouchard, T. J., Jr. (1997). Genetic influence on mental abilities, personality, vocational interests, and work attitudes. International Review of Industrial and Organizational Psychology, 12, 373–395.
- Carroll, J. B. (1993). Human cognitive abilities: A survey of factor-analytic studies. Cambridge, England: Cambridge University Press.
- Ceci, S. J., & Williams, W. M. (2011). Understanding current causes of women’s underrepresentation in science. Proceedings of the National Academy of Sciences, 108, 3157–3162.
- Corno, L., Cronbach, L. J., et al. (Eds.) (2002). Remaking the concept of aptitude: Extending the legacy of Richard E. Snow. Mahwah, NJ: Erlbaum.
- Dawis, R. V. (1992). The individual differences tradition in counseling psychology. Journal of Counseling Psychology, 39, 7–19.
- Dawis, R. V. (1991). Vocational interests, values and preferences. In M. D. Dunnette & L. M. Hough (Eds.), Handbook of industrial & organizational psychology (2nd ed., Vol. 2, pp. 833–872). Palo Alto, CA: Consulting Psychologists Press.
- Dawis, R. V., & Lofquist, L. H. (1984). A psychological theory of work adjustment. Minneapolis, MN: University of Minnesota Press.
- Day, S. X., & Rounds, J. (1998). Universality of vocational interest structure among racial and ethnic minorities. American Psychologist, 53, 728–736.
- Ferriman, K., Lubinski, D., & Benbow, C. P. (2009). Work preferences, life values, and personal views of top math/science graduate students and the profoundly gifted: Developmental changes and sex differences during emerging adulthood and parenthood. Journal of Personality and Social Psychology, 97, 517–532.
- Frey, M. C., & Detterman, D. K. (2004). Scholastic assessment or g? The relationship between the Scholastic Assessment Test and general cognitive ability. Psychological Science, 15, 373–378.
- Galton, F. (1869). Hereditary genius: An inquiry into its laws and consequences. New York, NY: Appleton.
- Gladwell, M. (2008). Outliers: The story of success. New York, NY: Little, Brown.
- Gottfredson, L. S. (2003). The challenge and promise of cognitive career assessment. Journal of Career Assessment, 11, 115–135.
- Gottfredson, L. S. (1997). Intelligence and social policy [Special issue]. Intelligence, 24(1).
- Guilford, J. P., Christensen, P. R., Bond, N. A., & Sutton, M. A. (1954). A factor analytic study of human interests. Psychological Monographs, 68(4, whole No. 35).
- Guttman, L. (1954). A new approach to factor analysis: The radex. In P. Lazarfield (Ed.), Mathematical thinking in the social sciences (pp. 258–348). Glencoe, IL: Free Press.
- Hilgard, E. R. (1980). The trilogy of mind: Cognition, affect, and conation. Journal of the History of the Behavioral Sciences, 16, 107–117.
- Holland, J. L. (1996). Exploring careers with a typology: What we have learned and some new directions. American Psychologist, 51, 397–406.
- Holland, J. L. (1959). A theory of vocational choice. Journal of Counseling Psychology, 6, 35–45.
- Hull, C. L. (1928). Aptitude testing. Chicago, IL: World Book Company.
- Humphreys, L. G., Lubinski, D., & Yao, G. (1993). Utility of predicting group membership and the role of spatial visualization in becoming an engineer, physical scientist, or artist. Journal of Applied Psychology, 78, 250–261.
- Hunt, E. (2011). Human intelligence. Cambridge, England: Cambridge University Press.
- Jensen, A.R. (1998). The g factor. Westport, CT: Praeger.
- Katzell, R. A. (1994). Contemporary meta-trends in industrial and organizational psychology. In H. C. Triandis, M. D. Dunnette & L. M. Hough (Eds.), Handbook of industrial/organizational psychology (2nd ed., Vol. 4, pp. 1–89). Palo Alto, CA: Consulting Psychology Press.
- Kell, H. J., Lubinski, D., & Benbow, C. P. (2013a). Who rises to the top? Early indicators. Psychological Science, 24, 648-659.
- Kell, H. J., Lubinski, D., Benbow, C. P., & Steiger, J. H. (2013b). Creativity and technical innovation: Spatial ability’s unique role. Psychological Science, 24, 1831-1836.
- Lubinski, D. (2009). Exceptional cognitive ability: The phenotype. Behavior Genetics, 39, 350–358.
- Lubinski, D. (2004). Introduction to the special section on cognitive abilities: 100 years after Spearman’s (1904) “‘General intelligence,’ objectively determined and measured.” Journal of Personality and Social Psychology, 86, 96–111.
- Lubinski, D. (2000). Scientific and social significance of assessing individual differences: “Sinking shafts at a few critical points.” Annual Review of Psychology, 51, 405–444.
- Lubinski, D. (1996). Applied individual differences research and its quantitative methods. Psychology, Public Policy, and Law, 2, 187–203.
- Lubinski, D., & Benbow, C. P. (2006). Study of mathematically precocious youth after 35 years: Uncovering antecedents for the development of math–science expertise. Perspectives on Psychological Science, 1, 316–345.
- Lubinski, D., & Benbow, C. P. (2000). States of excellence. American Psychologist, 55, 137–150.
- Lubinski, D., & Dawis, R. V. (1992). Aptitudes, skills, and proficiencies. In M. D. Dunnette & L. M. Hough (Eds.), The handbook of industrial/organizational psychology (2nd ed., pp. 1–59). Palo Alto, CA: Consulting Psychologists Press.
- Lubinski, D., & Humphreys, L. G. (1997). Incorporating general intelligence into epidemiology and the social sciences. Intelligence, 24, 159–201.
- Lubinski, D., Benbow, C. P., Shea, D. L., Eftekhari-Sanjani, H., & Halvorson, M. B. J. (2001). Men and women at promise for scientific excellence: Similarity not dissimilarity. Psychological Science, 12, 309–317.
- Lubinski, D., Benbow, C. P., Shea, D. L., Eftekhari-Sanjani, H., & Halvorson, M. B. J. (2001). Men and women at promise for scientific excellence: Similarity not dissimilarity. Psychological Science, 12, 309–317.
- Lubinski, D., Benbow, C. P., Webb, R. M., & Bleske-Rechek, A. (2006). Tracking exceptional human capital over two decades. Psychological Science, 17, 194–199.
- Park, G., Lubinski, D., & Benbow, C. P. (2008). Ability differences among people who have commensurate degrees matter for scientific creativity. Psychological Science, 19, 957–961.
- Park, G., Lubinski, D., & Benbow, C. P. (2007). Contrasting intellectual patterns for creativity in the arts and sciences: Tracking intellectually precocious youth over 25 years. Psychological Science, 18, 948–952.
- Prediger, D. J. (1982). Dimensions underlying Holland’s hexagon: Missing link between interests and occupations? Journal of Vocational Behavior, 21, 259–287.
- Rounds, J. B., & Tracey, T. J. (1990). From trait-and-factor to person–environment fit counseling: Theory and process. In W. B. Walsh & S. H. Osipow (Eds.), Career counseling: Contemporary topics in vocational psychology (pp. 1–44). Hillsdale, NJ: Erlbaum.
- Savickas, M. L., & Spokane, A. R. (1999). Vocational interests: Meaning, measurement, and counseling use. Pale Alto, CA: Davies-Black/Counseling Psychologists Press.
- Scarr, S. (1996). How people make their own environments: Implications for parents and policy makers. Psychology, Public Policy, and Law, 2, 204–228.
- Scarr, S., & McCartney, K. (1983). How people make their own environments: A theory of genotype → environment effects. Child Development, 54, 424–435.
- Schmidt, D. B., Lubinski, D., & Benbow, C. P. (1998). Validity of assessing educational–vocational preference dimensions among intellectually talented 13-year-olds. Journal of Counseling Psychology, 45, 436–453.
- Schmidt, F. L., & Hunter, J. E. (1998). The validity and utility of selection methods in personnel psychology: Practical and theoretical implications of 85 years of research findings. Psychological Bulletin, 124, 262–274.
- Simonton, D. (1994). Greatness: Who makes history and why. New York, NY: Guilford Press.
- Snow, R. E., & Lohman, D. F. (1989). Implications of cognitive psychology for educational measurement. In R. L. Linn (Ed.), Educational measurement (3rd ed., pp. 263–332). New York, NY: Macmillan.
- Snow, R. E., Corno, L., & Jackson, D., III. (1996). Individual differences in affective and conative functions. In D. C. Berliner & R. C. Calfee (Eds.), Handbook of educational psychology (pp. 243–310). New York, NY: Macmillan.
- Spearman, C. (1904). “General intelligence,” objectively determined and measured. American Journal of Psychology, 15, 201–292.
- Strong, E. K., Jr. (1943). Vocational interests for men and women. Stanford, CA: Stanford University Press.
- Su, R., Rounds, J., & Armstrong, P. I. (2009). Men and things, women and people: A meta-analysis of sex differences in interests. Psychological Bulletin, 35, 859–884.
- Wai, J., Lubinski, D., & Benbow, C. P. (2009). Spatial ability for STEM domains: Aligning over fifty years of cumulative psychological knowledge solidifies its importance. Journal of Educational Psychology, 101, 817–835.
- Webb, E. (1915). Character and intelligence. British Journal of Psychology, monograph supplement III.
- Webb, R. M., Lubinski, D., & Benbow, C. P. (2007). Spatial ability: A neglected dimension in talent searches for intellectually precocious youth. Journal of Educational Psychology, 99, 397–420.
- Wilson, E. O. (1998). Consilience: The unity of knowledge. New York, NY: Knopf.
Authors
- David LubinskiDavid Lubinski is a Professor of Psychology at Vanderbilt University where he co-directs the Study of Mathematically Precocious Youth – a planned 50-year study of over 5,000 intellectual talented participants (identified during adolescence), currently in its fourth decade. He has received APA’s Early Career Award in Psychometrics/Applied Research, and serves as President of the International Society for Intelligence Research (ISIR) and the Board of Trust for the Society for Multivariate Experimental Psychology (SMEP).
Creative Commons License



